Contents of data management plans and RDM proposal chapters
Explanations and examples
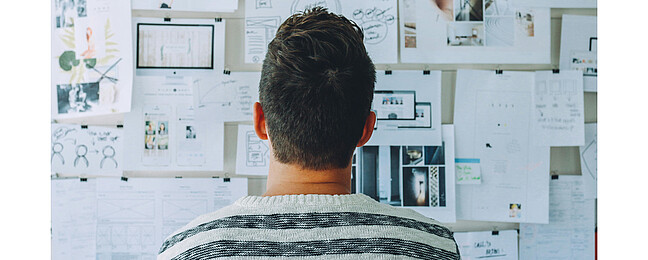
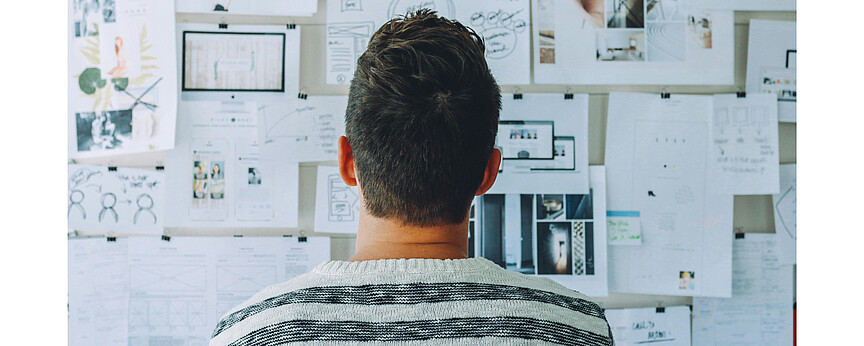
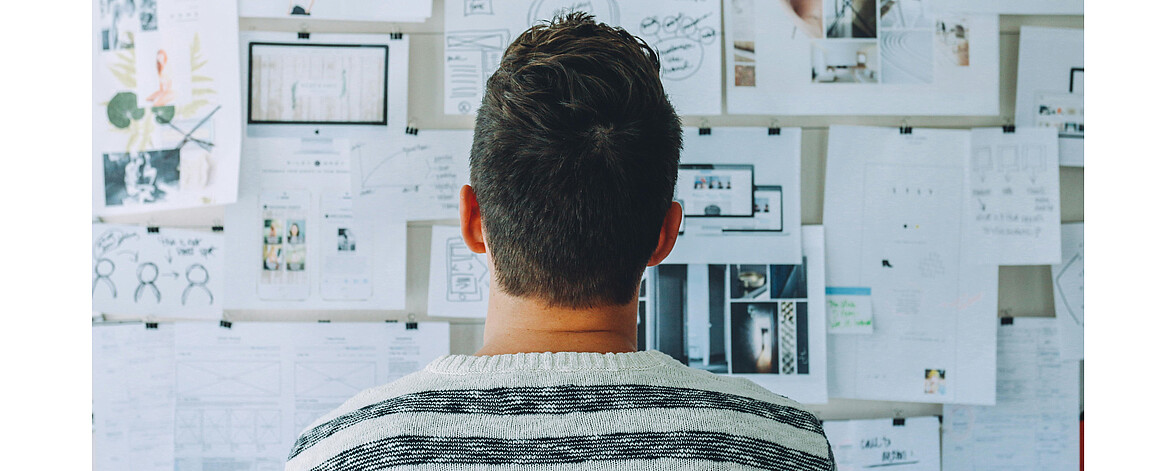
On this page, we would like to provide you with recommendations for content and examples of wording in data management plans and proposal chapters on research data management. When drafting a corresponding text, you should name the services and infrastructures you wish to use as specifically as possible. On the ‘Tools’ subpage, we have described and linked to a number of tools that are available to you at LUH/TIB and beyond.
The structure of this page is based on the structure of the DFG checklist for the handling of research data. However, the contents are relevant for any type of data management plan or RDM proposal chapter, regardless of the formal requirements of a specific funding organisation.
Below each of the six chapters, you will find fictitious text examples to give you an idea of how statements could be formulated in a DMP or proposal chapter. These texts are completely made up and no good as copy templates. Each project has individual framework conditions, resources, method sets and research objectives, and data management must be adapted accordingly. Standardized text modules are therefore not feasible. Please feel free to use our counselling service if you need support with the design or description of data management in your project.
1. Data description
-
How does your project create new data? Are existing data reused?
Describe the tools and methods you use to collate existing data or generate new data. Tools can be specific software tools or perhaps simply a notebook. Methods could include, for example, measurements, observations, simulations, surveys, interviews, archive research or coding.
-
Which data types, in terms of data formats, are created in your project, and how are they processed?
Name the formats of both, raw data and, if applicable, derived data products or converted versions here. For example, scanned documents could initially be in tiff format, but then saved in pdf format after an automatic text recognition. Measurement data is often put out in proprietary formats from the manufacturer of the measuring device, but can then be converted into formats such as csv if necessary.
-
What is the expected volume of this data?
This estimate is important in order to be able to clarify in good time whether you have sufficient storage capacity and bandwidth for data transfers at your disposal. The decisive factor here is the maximum amount of data stored simultaneously. You should hence also consider backup copies and temporary files that may be created as an intermediate step during processing. If in doubt, calculate generously.
Relevant tools and services
Fictional examples
-
Quantitative data derived from an online survey
We conduct surveys using the online tool Limesurvey. The results are first exported into the csv format and then imported into Stata for further statistical analysis and saved in the dta format. For the archiving purposes after the analysis has been completed, the data is exported back into csv format and saved together with a do-file. The data volume should be less than 5 GB.
-
Sensor data from controlled laboratory experiments
The project generates video and measurement data from experiments. A high-speed camera delivers 2000 images per second. Each recording lasts approx. 6 seconds and requires approx. 72 GB of storage space. We reckon with around 100 recordings (approx. 7.2 TB). The raw data is of the manufacturer-specific CINE format, but is converted to the AVI format before the data is archived. In addition, radar measurement data in the binary format MDF4 is generated, the total volume of which should not exceed 200 GB.
-
Development of software and simulation data
During the development of the digital twin, mainly self-programmed Python code is created. The simulations carried out using this code are based on standardized property values for metal materials (EN/DIN). The output is data in the NetCDF format as well as static graphical representations as two- and three-dimensional diagrams and time series as PDF or image files (JPG/PNG). Depending on the parameters set, between 5 and 20 GB of data are likely to be generated per simulation run. The results of test simulations in the development phase are not saved permanently. Therefore, a maximum of 500 GB of data accrue at any one time.
2. Documentation and data quality
-
What approaches are being taken to describe the data in a comprehensible manner (such as the use of available metadata, documentation standards or ontologies)?
The generation of new data or the reuse of existing data in your projects should be documented as soon as it occurs. This way it is always clear from where the data originates from or how it was created. Each subsequent processing step should also be recorded. It is advisable to use structured database recording systems instead of pure continuous text descriptions in order to be able to search efficiently and reliably for specific information in this documentation at a later date.
There are standards for recording such metadata that define the exact format for certain information (e.g. authors, survey date, project context, etc.). The naming of other fields and categories that are not predefined in such a metadata schema should be based on subject-specific standard vocabularies (terminologies and ontologies), if these exist. This way, you increase comparability and interoperability with data of a similar nature from other projects.
-
What measures are being adopted to ensure high data quality? Are quality controls in place and if so, how do they operate?
There are many potential sources of error when collecting and processing data, such as incorrectly calibrated measuring devices, transmission errors, corrupt files and all conceivable forms of human error. As incorrect data also leads to incorrect research results, regular quality checking mechanisms are important. Depending on the type of data, these measures can sometimes be (partially) automated. For example, creating and comparing checksums helps to recognize files that have been damaged or unintentionally changed during copying. Programmes such as Excel are known to change character encodings and number formats without prompting, which is why a comparison between the original and the Excel version is recommended when converting. If possible, transcriptions and annotations should always be checked independently by at least two persons.
-
Which digital methods and tools (e.g. software) are required to use the data?
In principle, scientific data should be made available in such a way that it can be read with standard software. Proprietary data formats that can only be read with special and possibly cost-intensive software should therefore additionally be converted into open formats if possible, even if it sometimes results in a reduced functionality. At the very least, however, it should always be specified exactly which version of a programme and, if applicable, also which operating system is required.
Relevant tools and services
Fictional examples
-
Annotated transcripts
The interviews are processed with the free software EXMARaLDA according to the standards of the ‘Gesprächsanalytisches Transkriptionssystem 2’ (GAT2) and provided with a corresponding header. After the automatic, AI-supported (OpenAI Whisper) transcription of the interview recordings, a quality check is carried out by one of the project participants. The audio recording is played back, and the transcript is read at the same time. Incorrectly transcribed content is corrected manually. In addition, pauses and non-speech sounds such as laughter, coughing, clearing of the throat, etc. are recognized. The finished transcripts are additionally converted into the RTF format, which can be read with any standard word processing programme.
-
CAD data
All components are designed digitally with SOLIDWORKS. The industrial partner provides an instance of SOLIDWORKS Product Data Management (PDM), in which all data is versioned and its processing history documented. SOLIDWORKS Simulation is used to simulate the mechanical stability of each component to identify incorrect calculations before a physical prototype is produced. At the end of the project, the documentation for each component is exported as a static PDF file and the CAD file itself is exported into the STEP format (ISO 10303).
-
Geo data
Vector data is of the shp format, raster data of the Geotiff or csv formats. A PostGIS geodatabase is created additionally. These formats can be integrated into any standard geographic information system. Vector data is checked for obvious geometric inconsistencies using the QGIS plug-in ‘Geometry Checker’ and corrected if necessary. All metadata is recorded in accordance with ISO standard 19115. Methods for processing and analysing the geodata are described in the project wiki (Doku-Wiki), which is included in the scope of the LUIS Projektablage.
3. Storage and technical archiving during the project
-
How is the data to be stored and archived throughout the project duration?
Explain that you will have sufficient storage capacity at your disposal. If available, use professionally maintained servers provided by your institute or the central IT services and find out whether and how these are backed up. If your institute uses the LUIS Backup & Restore service, a daily backup of the institute's servers will be created automatically. Otherwise, see for an equivalent backup mechanism yourself. The copy should always be in a different location, i.e. at least in a different building. For very large data volumes, also check whether the bandwidth is sufficient for daily transfer to a backup server. Data that is stored exclusively on local hard drives or external storage media runs a high risk of loss, e.g. due to technical defects, damage or theft of the storage media.
-
What is in place to secure sensitive data throughout the project duration (access and usage rights)?
Data is considered sensitive in particular if the rights of third parties could be violated by unauthorized access or data leakage. This is generally the case with personal data, but also with data that has been made available to you on the condition of confidentiality or under special terms of use, for example by project partners from the industry or by commercial data providers. Ethical aspects may also play a role. The more severe the consequences of theft or unauthorized access to data would be, the better the data should be protected. If you work with such data, you should take technical measures to ensure that only authorized persons have access to it. Typical measures include encrypting storage media, setting up a differentiated access management, keeping storage media in specially secured rooms and, if necessary, complete disconnection from the internet and intranet (air gap).
Relevant tools and services
Fictional examples
-
Sensitive qualitative interview data
Voice recorders with an encryption function are used to record the interviews. Immediately after the interviews are completed, these audio files are copied to the partition of the institute's server reserved for the project and then deleted from the recording device. The entire partition is encrypted according to the AES-256 standard. All persons involved in the project have their own encrypted folder within this area, which can only be opened with a personal password. This means that only encrypted files are transferred during the daily backup of the institute's server via the LUIS backup & restore service.
-
Technical data relevant for patents
In accordance with the confidentiality agreement, all construction data is stored on a server of the industry partner. Access is only possible online via a VPN tunnel and after prior authorization with username and password. Less sensitive data related to the experiments at the institute are stored on an encrypted partition of the institute's server reserved for the project. All project participants have the same access and editing rights to this data.
-
Data from the public domain
All data is stored in the cloud storage of a "Projektablage" set up by LUIS (Projekt-Seafile). The cloud servers are automatically backed up daily by LUIS. Only publicly accessible and copyright-free data is collected and processed in the project. The data newly created in the scope of the project will also be published under a public domain mark. Special protection against unauthorized access is therefore not required.
4. Legal obligations and conditions
-
What are the legal specifics associated with the handling of research data in your project?
When handling research data, often affected areas of law are data protection and personal rights as well as copyright and patent law. In order to avoid any violations of law, check whether and which legal standards or existing contractual provisions may be affected before reusing, collecting, processing, evaluating and, if applicable, archiving and publishing data. Pay particular attention to license conditions, declarations of consent, non-disclosure agreements, contracts for commissioned processing and cooperation agreements. If necessary, make contractual agreements yourself. When reviewing existing legal texts as well as when drawing up new contracts, you should always consult legal experts!
-
Do you anticipate any implications or restrictions regarding subsequent publication or accessibility?
In principle, most major funding organizations now expect data from the projects they fund to be made publicly available for subsequent use, provided there are no legal or ethical reasons to the contrary. However, access to data must always be restricted or even completely denied if third-party rights would otherwise be violated. This is regularly the case with personal data, for example, unless the data subjects have given their legally valid consent to publication. Non-disclosure agreements with (raw) data providers, certain licence conditions, commercial interests or ethical considerations can also rule out publication or restrict access. If you generate new data collaboratively, all parties involved should agree to the planned publication. It is therefore advisable to reach written agreements before starting a project.
-
What is in place to consider aspects of use and copyright law as well as ownership issues?
In this context, it is particularly important to consistently document the sources or the generation of data as well as licence conditions and rights holders, if applicable. If you want to process data from external sources for which no precise licence conditions or other re-use agreements are known, obtain all necessary usage rights from the rights holders in writing before using the data. Reach contractual agreements within your project team on the extent to which data generated in the project may be used by all participants. Also take into account the possibility of participants leaving the project prematurely or changing employers. If you publish data yourself, attach a licence with unambiguous and legally binding conditions for re-use.
-
Are there any significant research codes or professional standards to be taken into account?
A large part of the ‘Guidelines for Safeguarding Good Research Practice’ refers to the handling of research data. More specific requirements may be laid down in discipline-specific guidelines and codes, but these are not always widely known. In any case, check whether documents relevant to your subject exist whose contents should be taken into account. The DFG has compiled subject-specific recommendations on the handling of research data, most of which have been published by the review boards or major research associations. On forschungsdaten.info there is a subpage on RDM guidelines, including discipline-specific guidelines. Another compilation can be found at forschungsdaten.org (German only). Many NFDI consortia now offer helpdesks where you can also enquire about current standards relevant to your discipline.
Relevant tools and services
Fictional examples
-
Compilation of text corpora
The project compiles and analyses excerpts from published texts as well as unpublished archive material. A Zotero literature database will be used to systematically record the sources, copyright holders and usage conditions for all texts. An expert legal examination concluded that the use of the published texts in the form envisaged in this project is covered by the copyright limitation provisions. In contrast, the conditions for the use of the unpublished material vary from archive to archive and other rights holders, in particular the authors or their heirs. Attempts will be made to reach contractual agreements with the respective parties that not only permit the evaluation but also the publication of these texts, possibly in a modified form (e.g. anonymised or paraphrased).
-
Animal experiments on mice
Experiments on mice are indispensable for the realization of the project. A corresponding authorization will be applied for from the Lower Saxony State Office for Consumer Protection and Food Safety. For ethical reasons, we limit the number of experiments and the animals used in them to the absolutely necessary minimum in accordance with the 3R principles. The experiments are designed in such a way that the animals are exposed to as little stress and pain as possible. The total of 20 animals required from the patented breeding line C57BL/6J are used and kept in accordance with the licence conditions of TACONIC BIOSCIENCES.
-
Prototype containing externaly developed technical components
In accordance with the funding conditions for this tender, the construction plans for the innovative wind turbine developed in the project are published under open licences such as MIT or CC0 and can therefore also be used commercially free of charge. However, some of the installed components are provided by external manufacturers and subject to their licence terms. The exact circuit diagrams for these as yet unpatented parts were made available to the project by the manufacturers under the condition of confidentiality and non-disclosure. The plans and technical descriptions intended for publication therefore only represent these components as a whole and without details of the internal structure. Furthermore, these documents will be submitted to the external manufacturers for review prior to publication.
5. Data exchange and long-term data accessibility
-
Which data sets are especially suitable for use in other contexts?
A reuse of data is particularly common in follow-up projects in which the staff composition is similar to that of the projects in which they were generated. Other reuse scenarios are third-party research projects with a similar topic. However, you should also consider the possibility of reusing data in comparative overview studies or in completely different contexts, for example for training an AI model. For such scenarios in particular, it is important that your data are available in standard formats that are as generic, open and machine-readable as possible and that they are documented in such a way that their structure and origin can also be understood by non-specialists.
-
Which criteria are used to select research data to make it available for subsequent use by others?
Provided there are no legal or ethical reasons against making data available for re-use, the following criteria could be relevant:
- The data is needed to independently verify and reproduce published research results (good research practice).
- The data is unique and could not be recorded again in the same form.
- The data may be of interest for further research projects (also from other disciplines) and could be usefully re-utilised by them.
- The data is quality-checked and well documented.
- Your research is based on data that you have received from other persons or projects, on the condition that you make your own data available for (free) re-use at a later date as well.
If in doubt, make all data available if possible, as long as it is legally permitted and, in terms of volume, technically and economically feasible.
-
Are you planning to archive your data in a suitable infrastructure? If so, how and where?
Data that is relevant for reproducing research results must generally be securely kept for at least ten years. Professional data archives in which data is stored redundantly and automatically checked for integrity (bitstream preservation) are ideal for this purpose. The storage media themselves are regularly renewed. This measure minimizes the risk of data loss due to defective storage media and corrupt files. The LUIS operates such a data archive, which can be used by all LUH employees. Data that has been published in a trustworthy repository does not necessarily require additional archiving, as such repositories should generally fulfil the same technical requirements as pure data archives. By contrast, standard external storage media such as hard drives, DVDs etc. are definitely not suitable for archiving. The media degenerate over time, which may result in corrupt and unreadable files.
-
Are there any retention periods? When is the research data available for use by third parties?
If the analysis of the data has not yet been completed after the end of the project, it may still be useful to already upload this data to a data repository, but to set an embargo (blocking period). In this case, public access is only possible after this period has expired. The advantage is that you no longer have to worry about publishing the data after the end of the project, as this is done automatically at the specified point in time. However, not all repositories offer the option to set an embargo. The period should usually not exceed one year.
Relevant tools and services
Fictional examples
-
Archiving of qualitative data
The audio recordings of the interviews are deleted at the end of the project, as they are difficult to anonymize and, in this case, offer no added value over the transcripts. The anonymized transcripts are archived at the Research Data Centre for Qualitative Social Science Research Data (Qualiservice). In accordance with the informed consent form signed by the data subjects, these data may only be used for research purposes. Qualiservice therefore acts as a data trustee and only provides the data after carefully verifying a plausible research interest and after having signed a contractual agreement.
-
Publication of simulation data
The code and algorithms required to reproduce the simulations are stored in GitHub repositories, which are archived in Zenodo. They are given a DOI, which in turn can be cited in scientific articles and on the project's website. The simulation results themselves are not kept, as they require a lot of storage space. If required, they can be reproduced quickly and easily using the code.
-
Publication with embargo
The observation data collected in the project are unique and not reproducible. In principle, they are suitable for reuse in future long-term and comparative studies. At the end of the project period, the project participants should therefore upload all the data their research is based on to an established disciplinary repository for publication under an open licence. If the analysis has not yet been completed by this time, they can place an embargo of no more than twelve months on the data. During this period, public access will not yet be possible.
6. Responsibilities and resources
-
Who is responsible for adequate handling of the research data (description of roles and responsibilities within the project)?
Ultimately, the respective management of a research project is responsible for the appropriate handling of research data. However, they can and should delegate tasks and responsibilities, for example to principal investigators, doctoral students or student assistants. Depending on the size of the project and the complexity of the data management, it may make sense to employ a person who takes on the role of a full-time data steward and dealing with the administration and documentation of the data, as well as for setting up and operating the necessary systems. The operators of storage infrastructures should guarantee their secure operation. External service providers should be obliged to maintain confidentiality and comply with security standards by means of a commissioned processing contract.
-
Which resources (costs; time or other) are required to implement adequate handling of research data within the project?
Data management mainly requires resources in terms of personnel, infrastructure and external services. Ander all circumstances, the corresponding costs should be realistically estimated from the outset, budgeted and, if necessary, applied for. A full-time data steward could, for example, correspond to a TVL-13 position. The calculation becomes more difficult if the data management is to be carried out entirely by the scientific staff, who then have to reserve part of their working time for this task. On the infrastructure side, the required storage space in the short and long term should be considered. In this respect, costs may not only increase due to volume, but also because of special requirements, for example in terms of security. Adequate infrastructure is not always sufficiently available within the university's basic facilities. External services can sometimes be used already at the stage of data acquisition and processing. However, costs are most frequently incurred for long-term archiving, curation and, if necessary, public provision of data in external archives and repositories.
-
Who is responsible for curating the data once the project has ended?
At the simplest level, curation means simple bitstream preservation, i.e. the guarantee that files remain unchanged. If the data is stored in a professional repository or data archive, this aspect falls under the responsibility of the relevant infrastructure operators. The project director should ensure that all relevant data is stored in such facilities at the end of the project. Active curation, on the other hand, may also include quality checks, enrichment and checking of metadata and regular conversion to newer file formats. Some repositories and archives offer such services (for a fee). Curation data long-term on your own is not advisable.
Relevant tools and services
Fictional examples
-
Joint project with data steward
The responsibilities for handling research data are set out in more detail in an internal guideline of the joint project. From among the PIs, Prof X is appointed the primary contact person on the topic for the funding body and external enquiries. We apply for a TV-L 13 full-time position for a data manager who will configure and maintain the systems for data and knowledge management jointly used by all partners and who will be available to all members of the network as a contact person for practical questions. The computer centre of University Y provides the technical infrastructure, including the backup servers, and is responsible for its technical functionality and security. The responsibility for the correct processing, documentation and storage of data at the different partner institutions lies with the respective sub-project managers and, regarding the technical operation, with the local infrastructure operators.
-
Junior research group, big data volume
The applicant is responsible for the data management in general. She will instruct the doctoral researchers in her junior research group and will randomly check during the course of the project whether they have named, filed and documented their data in accordance with the data management plan. Due to the expected data volume of approx. 5 TB, a fee must be paid for the use of the High-Seas cloud service, which is operated by LUIS. The fee will average around 500 euros per year and will therefore amount to around 2,000 euros over the four-year project duration. At the end of the project, the data will be published in the open and long-term readable file formats csv, tiff and png in the established disciplinary repository Pangaea, which is operated by the Alfred Wegener Institute (AWI). The AWI is therefore responsible for the long-term preservation and availability of the data. No curation of the data is required beyond a mere bitstream preservation.
-
One-person-project with external data curation
The applicant is responsible for the correct handling of the research data processed in the project in line with disciplinary, legal und technical standards. He carries out all processing and documentation steps himself and allocates approximately 10% of his weekly working time for this task. If needed, he may seek professional advice from the LUH central Team Research Data Management or the helpdesk of the NFDI consortium X. The IT administrator of the Institute Y is responsible for the technical operation and security of the institute's server used as storage location. The operation and security of the backup servers are ensured by LUIS. At the end of the project, a final quality check, long-term curation, and publication of the data will be conducted via the paid GESIS data service “Premium Archiving”. A corresponding tender amounting to XXX euros has been obtained.